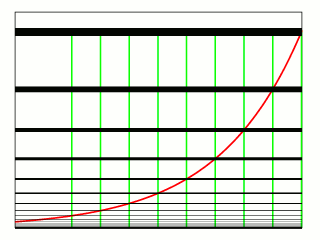
AI: AI Scaling Trifurcates and Accelerates. RTZ #641
It’s easy for regular folks to get jaded by words like exponential ‘AI Scaling’, when used and repeated by AI pundits and media endlessly in this AI Tech Wave.
And it’s hard to parse through the differences in LLM AI buzzwords and keep them straight in daily news inflows. Words like ‘pre and post-training’, ‘inference’, ‘test time compute’, ‘reinforcement learning’ with ‘human feedback’ (RLHF), and the renewed post DeepSeek popularity of ‘reinforcement learning (RL) (both supervised and unsupervised’, and of course ‘distillation’, which is about using bigger models both closed and open to train data for smaller models and their results. Most of these are in the legend below in the oft-quoted AI Tech Stack chart below:
I go through this key point despite having discussed the current transition of LLM AIs from chatbots to AI Reasoning to AI Agents on the way to AGI (artificial general intelligence).
And because it’s easy to under-appreciate the fundamental acceleration of these multiple AI Scaling definitions and new applications.
The WSJ lays it all out in “Why AI Spending Isn’t Slowing Down”:
“Soaring demand for reasoning models will consume electricity, microchips and data-center real estate for the foreseeable future.”
“Despite a brief period of investor doubt, money is pouring into artificial intelligence from big tech companies, national governments and venture capitalists at unprecedented levels. To understand why, it helps to appreciate the way that AI itself is changing.”
“The technology is shifting away from conventional large language models and toward reasoning models and AI agents. Training conventional large language models—the kind you’ve encountered in free versions of most AI chatbots—requires vast amounts of power and computing time. But we’re rapidly figuring out ways to reduce the amount of resources they need to run when a human calls on them. Reasoning models, which are based on large language models, are different in that their actual operation consumes many times more resources, in terms of both microchips and electricity.”
“Since OpenAI previewed its first reasoning model, called o1, in September, AI companies have been rushing to release systems that can compete. This includes DeepSeek’s R1, which rocked the AI world and the valuations of many tech and power companies at the beginning of this year, and Elon Musk’s xAI, which just debuted its Grok 3 reasoning model.”
And of course DeepSeek was the next big catalyst on redefining some of the AI words above, and showing new, efficient ways to use AI Compute in far more efficient ways.
“DeepSeek caused a panic of sorts because it showed that an AI model could be trained for a fraction of the cost of other models, something that could cut demand for data centers and expensive advanced chips. But what DeepSeek really did was push the AI industry even harder toward resource-intensive reasoning models, meaning that computing infrastructure is still very much needed.”
“Owing to their enhanced capabilities, these reasoning systems will likely soon become the default way that people use AI for many tasks. OpenAI Chief Executive Sam Altman said the next major upgrade to his company’s AI model will include advanced reasoning capabilities.”
“Why do reasoning models—and the products they’re a part of, like “deep research” tools and AI agents—need so much more power? The answer lies in how they work.”
This is what is easily missed with a firehose of AI words mentioned above, that AI Scaling split into other forms, all scaling faster from a lower, new base:
“AI reasoning models can easily use more than 100 times as much computing resources as conventional large language models, Nvidia’s vice president of product management for AI, Kari Briski, wrote in a recent blog post.”
This is the Nvidia chart to truly understand the ‘trifurcation’ of AI Scaling ahead:
“That multiplier comes from reasoning models spending minutes or even hours talking to themselves—not all of which the user sees—in a long “chain of thought.” The amount of computing resources used by a model is proportional to the number of words generated, so a reasoning model that generates 100 times as many words to answer a question will use that much more electricity and other resources.”
“Things can get even more resource-intensive when reasoning models access the internet, as Google’s, OpenAI’s and Perplexity’s “deep research” models do.”
Which is why I re-articulated that Nvidia and other AI infrastructure companies, were likely to see MORE demand for their products for AI data centers, not less.
“These demands for computing power are just the beginning. As a reflection of that, Google, Microsoft and Meta Platforms are collectively planning to spend at least $215 billion on capital expenditures—much of that for AI data centers—in 2025. That would represent a 45% increase in their capital spending from last year.”
Even these new ways ‘Scale AI’ are just the beginning of the beginning:
“This is just the starting point. As businesses are discovering that the new AI models are more capable, they’re calling on them more and more often. This is shifting demand for computing capacity from training models toward using them—or what’s called “inference” in the AI industry.’“
“All of the big AI labs at companies like OpenAI, Google and Meta are still trying to best one another by training ever-more-capable AI models. Whatever the cost, the prize is capturing as much of the still-nascent market for AIs as possible.”
“Over the next couple of years, new innovations and more AI-specific microchips could mean systems that deliver AI to end customers become a thousand times more efficient than they are today, says Tomasz Tunguz, a venture capitalist and founder of Theory Ventures. The bet that investors and big tech companies are making, he adds, is that over the course of the coming decade, the amount of demand for AI models could go up by a factor of a trillion or more, thanks to reasoning models and rapid adoption.”
The key ithing to understand is how more variable AI interactions drive more AI Intelligence tokens generated for input and output via ever scaling AI compute, and this is important, at ever lower costs:
“Every keystroke in your keyboard, or every phoneme you utter into a microphone, will be transcribed or manipulated by at least one AI,” says Tunguz. And if that’s the case, he adds, the AI market could soon be 1,000 times larger than it is today.”
And that is the most important thing to understand about this AI Tech Wave at this early stage. We’ve barely figured out how to make AI Scaling really Scale with the unprecedented amounts of AI Compute being built. And it’s not be enough for what’s going to be needed ahead. Stay tuned.
(NOTE: The discussions here are for information purposes only, and not meant as investment advice at any time. Thanks for joining us here)