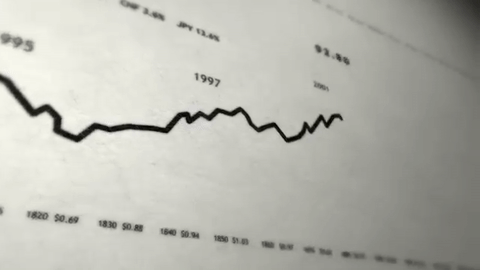
AI: Evaluating rapidly changing AI business models. RTZ #678
A few weeks ago I discussed how some AI startups are anecdotally scaling faster to $100 million in annualized revenue run rates (ARRs) than comparable startups in previous tech waves.
How AI technologies are allowing them to do more with less. In particular enabling higher productivity with fewer people. And benefiting from the higher than normal experimentation by companies and developers worldwide to experiment with rapidly changing AI technologies.
And how DeepSeek was the most recent catalyst to these trends.
That trend seems to be continuing with some new data out, especially for startups in the upper tiers of the tech value stack below. Specifically in the boxes 4-6 including applications and services.
The Information provides new context in “The Valuation Puzzle of Post-Training AI Startups”:
“We’re starting to see more generative artificial intelligence-related startups announce they’ve crossed $100 million in annualized revenue. According to our Generative AI Database, at least 15 such startups have reached that threshold.”
“The latest is Mercor, which helps OpenAI and other AI developers find contractors to help train new models. They are often experts in subjects like software engineering, law and medicine and help improve AI answers about those fields by rating the AI’s answers or teaching the model a better approach.
This company would span Box 4 and 5 of the AI Tech Stack chart above.
“Mercor CEO Brendan Foody said on X last month that the company was generating $100 million in annualized revenue—typically meaning the past month’s revenue multiplied by 12—up from $1 million in ARR 11 months earlier. That kind of growth likely helped the company raise financing at a $2 billion valuation last fall.”
But these revenue rates all need to be examined a bit closer for how they’re structured, and their sustainability. And of course where these companies are in the evolution of AI services from chatbots to reasoning to agents and beyond:
“On the surface, that sounds impressive—and it is! But if there’s one thing we’ve learned in the AI boom, it’s that you shouldn’t treat the revenue of AI startups today the same way you would have treated enterprise tech revenue in the past.”
“For starters, Mercor pays out 60% to 70% of its top line revenue to the contractors, a current investor told me. That means that its net revenue is far less than half of the $100 million figure it announced.”
It’s a kind of detail that grew a metric like ‘Gross Merchandise Value’ or GMV, for ecommerce marketplaces like eBay and others over 25 years ago. (I helped take eBay public in 1998).
And the importance of separating out the net revenues to the company in question.
And of course identifying the operating margin characteristics of the core business:
“On a positive note, Foody said in the same post that Mercor “profited $1M+ last month.” The profit he’s referring to is operating profit, according to a person with direct knowledge of the company’s financials, and Mercor’s gross profit margin has also improved as the company has charged higher prices for contractors with deep expertise in their fields.”
These issues also apply to larger, more established companies like Scale AI, which is one of the leading companies in Box 4 above.
“While Mercor has a slightly different business model than bigger competitors like Scale AI ($1 billion in projected revenue last year), Turing ($300 million ARR as of the end of 2024) and Invisible ($134 million in revenue last year), they all face similar pressure on their gross margins. Last fall, for instance, we reported that Scale, valued at $13.8 billion, spends more than 50% of its revenue on the direct costs of its business, including contractor salaries.”
“The big difference between Mercor’s gross revenue and net revenue shouldn’t be a surprise for those who follow the sector in which it operates, also known as data labeling or post-training.”
And that’s why its important not to judge AI companies quickly on the raw ‘price to revenue’ metrics. Those are last resort valuation methodologies that professional analysts like me had to resort to in the earliest days of internet commercialization in the 1990s, when there was a paucity of other financial metrics.
It’s again an important consideration in the AI Tech Wave too:
“But these dynamics may be a surprise for those of you paying attention to valuation multiples of AI companies. Mercor’s multiple was around 100 times its gross annualized revenue of about $20 million when its last financing round was priced, according to the person with knowledge of its financials. That falls on the higher end of the spectrum, and in spite of not having a recurring revenue model.”
“Putting too much emphasis on the exact revenue multiple of early-stage startups is a losing game, of course. A lot of the time, investors are rewarding startups for how quickly they’re growing or how big they think the companies can get. Bidding wars among investors can also drive up prices.”
And it’s important to understand the rapid changes in these evolution of these startups’ nascent business models:
“But as AI models improve, it could get more difficult and expensive for these post-training firms to find the top experts in various fields. Their margins could potentially get hurt if they don’t charge higher prices for providing the contractors or find a cheaper or faster way for them to do their work.”
“Turing, Scale and Invisible have consulting businesses to fall back on as they work more closely with businesses to use the AI models their contractors have perfected. There are also new areas like data labeling for robotics, which Scale is getting into.”
And to keep an eye on the next phases of their businesses these early companies are trying to build and jump to:
“Mercor’s long-term plan, according to a blog post by Foody, is to expand its customers beyond AI firms to providing contractors to other businesses. Theoretically, these contractors could help businesses with short-term work like consulting projects or social media campaigns. Mercor hopes to use its work with AI labs to better predict how an individual contractor will perform on the job and how to match them with other types of business customers.”
As was discussed almost a decade ago on the topic of valuing ARRs:
“One artifact of the current market shift is the value of recurring revenue. What a dollar of ARR was worth before is not what it is worth now. That means that private companies valued some time ago on their recurring revenue, and recurring revenue growth may be in for a surprise when they seek new capital.”
“It’s easy to see the change in valuation in public companies that are mostly valued on their ARR. It’s a bit harder to see in private companies, given that fundraising cycles are far longer than the daily trading periods public-market analogs deal with. So, to that effect, we’ll start public and then go private.”
The point of this discussion is that early tech startups, especially in this AI Tech Wave, are still undergoing rapid, unpredictable, Cambrian explosions and evolutions of their business models. It’s why Wall Street has intense debates on these subjects both at the macro tech trend and invididual sectors/company level.
Relying on the snapshots of their financial metrics have to be done with an eye to the underlying sustainability and volatility, with appropriately adjusted risk premiums at this stage of this AI Tech Wave. Stay tuned.
(NOTE: The discussions here are for information purposes only, and not meant as investment advice at any time. Thanks for joining us here)