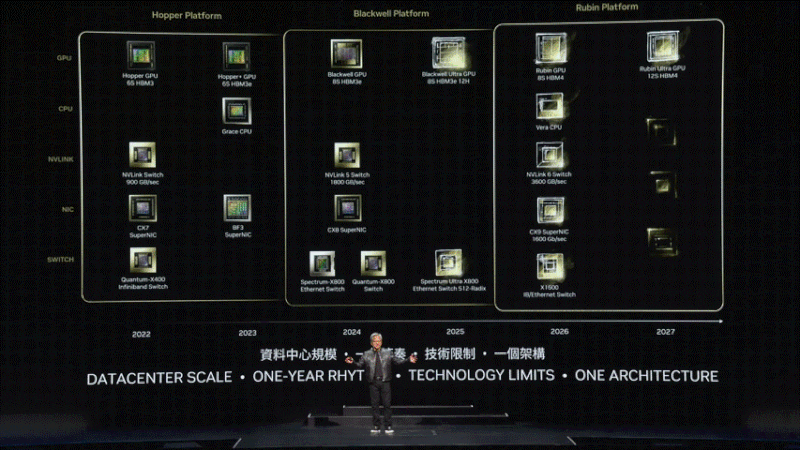
AI: Nvidia GTC 2025 geared for tech geeks. RTZ #663
Nvidia kicked off GTC 2025 (GPU Technology Conference) with a ‘Superbowl’ echoing bang, with most of the expected items I outlined yesterday. Nvidia founder/CEO Jensen Huang did an impressive job of presenting the extraordinary technical multi-year roadmap that the company has forged with its wide and deep set of partners to ramp up ‘AI factories’ that are energy optimized to produce ‘AI intelligence tokens’ at an AI Scale far beyond any of its competitors today.
The whole keynote is worth a full watch to take in the sheer technical immensity of what is being invented, and then AI Scaled up and out with a global set of partners. It’s a math and science heavy presentation, that will appeal to tech geeks more than financial market geeks.
Let’s discuss what was announced in terms of the roadmap and technical milestones before discussing the overall market related implications.
As Constellation summarizes it in “Nvidia launches Blackwell Ultra, Dynamo. outlines roadmap through 2027”:
“Nvidia launched Blackwell Ultra, which aims to boost training and test time inference, as the GPU giant makes the case that more efficient models such as DeepSeek still require its integrated AI factory stack of hardware and software.”
“The company also launched Dynamo, an open-source framework that disaggregates the AI reasoning process to optimize compute. For good measure, Nvidia laid out its plans for the next two years.”
The company used DeepSeek’s open source AI reasoning model as a core example to understand the AI Scaling math:
“In the leadup to Nvidia GTC, where Blackwell Ultra was announced by CEO Jensen Huang, has been interesting. The rise of DeepSeek and models that can efficiently reason at lower costs created some doubt about whether hyperscalers would need to spend heavily on Nvidia’s stack.”
“Nvidia launched Blackwell Ultra, which aims to boost training and test time inference, as the GPU giant makes the case that more efficient models such as DeepSeek still require its integrated AI factory stack of hardware and software.”
New was an operating system enviornment to build these AI factories at scale, dubbed ‘Dynamo’:
“The company also launched Dynamo, an open-source framework that disaggregates the AI reasoning process to optimize compute. For good measure, Nvidia laid out its plans for the next two years.”
“In the leadup to Nvidia GTC, where Blackwell Ultra was announced by CEO Jensen Huang, has been interesting. The rise of DeepSeek and models that can efficiently reason at lower costs created some doubt about whether hyperscalers would need to spend heavily on Nvidia’s stack.”
“Nvidia’s Huang in his keynote at Nvidia GTC made the case that Blackwell shipments are surging and that the insatiable demand for AI compute–and Nvidia’s stack–continues. Huang said Nvidia’s roadmap is focused on building out AI factories and laying out investments years in advance. “We don’t want to surprise you in May,” said Huang.”
And then Jensen went onto to discuss the AI roadmap for the next few years, with its ‘tick-tock’ upgrades:
“In a nutshell, Nvidia is sticking to its annual cadence, but sticking with the same chassis. The roadmap consists of the following:”
“Vera Rubin in second half of 2026.”
“Rubin Ultra in second half of 2027.”
“Huang said Nvidia’s annual cadence is about “scaling up, then scaling out.”
Key here is the company’s focus on the massive amount of networking innovation and scaling required to really use millions of these $30,000 per AI GPU chips in massively powered AI data centers:
“To that end, Huang noted that Nvidia’s roadmap will require bets on networking and photonics.”
And introduced their next AI chips after Blackwell and Rubin, with ‘Feynman’:
The whole roadmap needs to be understood in the context of the inference driven ‘trifurcation’ of AI Scaling Laws I’ve discussed earlier:
A technical summary of Blackwell is useful here:
“Key points about Blackwell Ultra include:”
-
“The platform is built on the Blackwell architecture launched a year ago.”
-
“Blackwell Ultra includes Nvidia GB300 NVL72 rack-scale system and the Nvidia HGX B300 NVL16 system.”
-
“Nvidia GB300 NVL72 connects 72 Blackwell Ultra GPUs and 36 Arm”
-
“Neoverse-based NVIDIA Grace CPUs. That setup enables AI models to tap into compute to come up with different solutions to problems and break down requests into steps.”
-
“GB300 NVL72 has 1.5x the performance of its predecessor.”
-
“Nvidia argued that Blackwell Ultra can increase the revenue opportunity of 50x for AI factories compared to Hopper.”
-
“GB300 NVL72 will be available on DGX Cloud, Nvidia’s managed AI platform.”
-
“Nvidia DGX SuperPOD with DGX GB300 systems use the GB300 NVL72 rack design as a turnkey architecture.”
“Blackwell Ultra is aimed at agentic AI, which will need to reason and act autonomously, and physical AI, which is critical to robotics and autonomous vehicles.”
He also went into how these innovations are critical for Nvidia’s cloud and neocloud partners in terms of their scaled up AI data centers being built for hundreds of billions and beyond:
“To scale out Blackwell Ultra, Nvidia said the platform will integrate with its Nvidia Spectrum-X Ethernet and Nvidia Quantum-X800 InfiniBand networking systems. Cisco, Dell Technologies, HPE, Lenovo and Supermicro are vendors that will offer Blackwell Ultra servers in addition to a bevy of contract equipment providers. Cloud hyperscalers AWS, Google Cloud, Microsoft Azure and Oracle Cloud Infrastructure will offer Blackwell Ultra instances along with specialized GPU providers such as CoreWeave, Crusoe, Nebius and others.”
“Nvidia is doubling down on its platform with Blackwell Ultra but also the the software and storage stack. At the same time Nvidia knows it has to fight to stay in the cloud data center as the cloud vendors are building out their inhouse AI platforms. Robotic automation – creating workloads for Nvidia is another strategy thar Huang and team are pursuing.
Huang threw chipmaking in a tizzy announcing Blackwell in a 1 year cycle from Hopper, unheard of in chip making. Nvidia has delivered.
The question is how do Nvidia’s plans stack up vis-a-vis the cloud vendors in-house plans. Do AWS and Microsoft have a chance? Does Nvidia cut into Google’s TPU lead? If Nvidia can have AWS and Microsoft give up building their custom chips it’s a mega win.”
Dynamo deserves some more technical context for those interested on both the technology and financial fronts:
“Dynamo: An open-source inference framework”
“Blackwell-powered systems will include Nvidia Dynamo, which is designed to scale up reasoning AI services. Nvidia Dynamo is designed to maximize token revenue generation and orchestrate and accelerate inference communication across GPUs. Huang said Dynamo is the “operating system of the AI factory.”
“Dynamo separates the processing and generation phases of large language models on different GPUs. Nvidia said Dynamo optimizes each phase to be independent and maximize resources.”
“Key points about Dynamo include:”
-
“Dynamo succeeds Nvidia Triton Inference Server.”
-
“By disaggregating workloads, Dynamo can double the performance of AI factories. Dynamo features a GPU planning engine, an LLM-aware router to minimize repeating results, low-latency communication library and a memory manager.”
-
“When running the DeepSeek-R1 model on a large cluster of GB200 NVL72 racks, Dynamo boosts the number of tokens by 30x per GPU.”
-
“Dynamo is fully open source and supports PyTorch, SGLang, Nvidia TensorRT-LLM and vLLM.”
-
“Dynamo maps the knowledge that inference systems hold in memory from serving prior requests (KV cache) across thousands of GPUs. It then routes new inference requests to GPUs that have the best match.”
In addition, the company had notable technical partnership announcements on self driving car partnerships with GM and others.
And a sharper focus on AI computers and devices locally, with dozens of PC partners around the world. It’s an area I’m excited about and have discussed at length.
Overall, that is quite the meaty technology roadmap for the next few years, and helpful for long-term followers of Nvidia.
And that’s ok for now that the key note still leaves the ongoing financial market concerns of how AI revenues and profits of its customers spending billions and soon trillions in AI infrastructure capex spend will continue to invest at this accelerating scale ahead of eventual customer market share, revenues and profits.
This is something I’ve discussed at length before, with the view that the financial metrics typically lag the secular technology innovations in every tech wave. And this AI Tech Wave is no different.
For long-term tech participants, there was much in what was outlined and announced to gain probabilistic confidence that Nvidia remains a formidable and potentially unique long-term beneficiary of this AI Tech Wave.
As Semianalysis summarizes in its technical analysis of Nvidia’s GTC announcements”:
”Soon after finishing his discussion on Blackwell, Jensen drove the point home by discussing how trated this by highlighting that Blackwell had an up to 68x performance gain over Hopper, resulting in an 87% decline in costs. Rubin is slated to drive even more performance gains – 900x that of Hopper, for a 99.97% reduction in cost.”
“Clearly, Nvidia is pursuing a relentless pace of improvement – as Jensen puts it: “When Blackwells start shipping in volume, you couldn’t even give Hoppers away”.
This is important to note as investors fret about competitors like AMD being able to compete with Nvidia’s roadmap and prices:
“Today, the Information published an article about Amazon pricing Trainium chips at 25% of the price of an H100. Meanwhile, Jensen is talking about “you cannot give away H100s for free after Blackwell ramps.” We believe that the latter statement is extremely powerful. Technology drives the cost of ownership, and everywhere we look (except for perhaps TPUs), we see copycats of Nvidia’s roadmap. Meanwhile, Jensen is driving what’s possible in technology.”
“New architecture, rack structures, algorithmic improvements, and CPO are each a technological differentiation between Nvidia and it’s competitors. Nvidia leads in almost all of them today, and when competitors catch up they push forward in another vector of progress. As Nvidia continues their annual cadence, we expect this to continue. There’s talk of ASICs being the future of compute, but we saw that a general platform that improves fast is hard to beat from the CPU era. Nvidia is recreating this platform again with GPUs, and we expect them to lead from the front.”
“Good luck keeping up with the Chief Revenue Destroyer.”
Nvidia is on an aggressive, multi-year AI GPU and Networking infrastructure roadmap ramp, that will be difficult for competitors to compete with in aggregate.
Stratechery makes the following key summarization point on Jensen’s presentation:
“The company has stepped up its cadence of new chips, and secondly, I think that the Dynamo vision is very compelling and — if it works — may cement Nvidia’s position in inference for much longer than expected. You want great companies to do great work, not rest on their laurels, and it’s good to know that Nvidia still seems capable of doing just that.”
The crux of Jensen’s keynote in my view, is how Nvidia’s hardware and software product roadmap is focused on ONE KEY EXTREME PROBLEM of the AI Tech Wave ahead of us. Depicted in the complex chart above.
It’s solving the provision of Inference at scale, optimized for input and output intelligence token provision balanced for ‘power optimized’ throughput and fast user response. All depending on the general purpose AI application being run at any given moment. Then driven through the ‘AI GPU Compute Factory’, at the optimum ‘ISO Power’ cost per intelligence token transaction.
Know that’s a lot of techno-speak. But they’re all important part of the daunting AI computing calculations ahead.
It’s the key extreme AI computing problem ahead at scale.
It’s why Jensen says at an important point in his keynote:
“So we’re not trying to sell you less, our sales guys are going, “Jensen, you’re selling them less” — this is better. And so anyways, the more you buy, the more you save. It’s even better than that.”
“Now, the more you buy, the more you make. Anyhow, remember everything is in the context of AI factories, and although we talk about the chips, you always start from scale up, the full scale up. What can you scale up to the maximum?”
Not just in terms of LLM AI, Small AI, and related areas on the software areas, but for the ‘physical AI’ markets that are developing around self driving cars, robots of every type from industrial to humanoids, and for AI served locally at the edge on computers and mobile devices. Nvidia has an impressive set of operating systems, hardware and software ecosystems, and an ever growing moat on those fronts.
But the nearer term focus of the financial markets on ‘where’s the financial beef’ will likely be an ongoing headwind. Especially in these complex political times of a US/China ‘AI space race’, trading curbs, tariffs, and de-globalisation in whatever form it ends up taking over the coming months.
It’s a complex landscape for Nvidia and the industry ahead, despite some of the most extraordinary technology innovations in this AI Tech Wave vs prior waves. For now, Jensen and team can take a well deserved bow for GTC 2025. Stay tuned.
(NOTE: The discussions here are for information purposes only, and not meant as investment advice at any time. Thanks for joining us here)