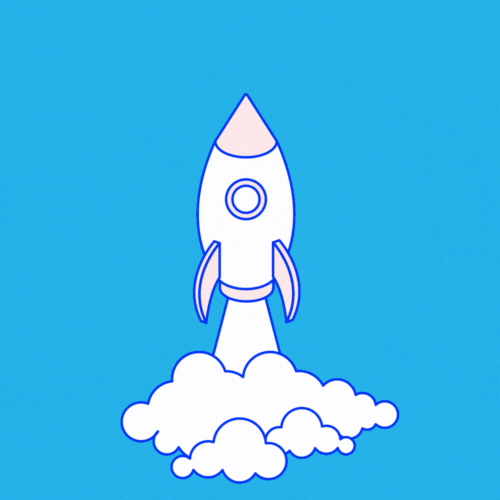
AI: 'Scaling AI' gets redefined (Part 2). RTZ #540
Couple of days ago I discussed the current popular topic that ‘AI Scaling’ at the big LLM AI companies like OpenAI and others, may be hitting some headwinds this AI Tech Wave.
This while the big tech companies are ramping up their historically unprecedented AI ‘Compute’ capex programs for AI GPUs, Data Centers, Power, and all the other inputs. All these and more are needed to make AI tasks like training, inference, synthetic data and content creation, as well as countless emerging AI applications do new ‘AI Reasoning and Agentic’ work at scale.
The Information this time looks at Google with the same question in “Following OpenAI, Google Changes Tack to Overcome AI Slowdown”:
“Google has recently struggled to achieve performance gains in its Gemini conversational artificial intelligence at the same rate it did last year, prompting researchers to focus on other ways to eke out gains, according to an employee who has been involved in the effort.”
“The situation appears similar to the slowdown in AI advances that rival OpenAI has experienced this year, which spurred the ChatGPT owner to use new techniques to overcome the challenge.”
“Google hasn’t achieved the performance gains some of its leaders were hoping for after dedicating larger amounts of computing power and training data—such as text and images from the web, this person said. Past versions of Google’s flagship Gemini large language model improved at a faster rate when researchers used more data and computing power to train them.”
I’ve of course discussed Google’s AI prospects and advantages with its AI assets including Gemini, vs its peers and competitors.
The Information continues:
“Google’s experience is another indication that a core assumption about how to improve models, known as scaling laws, is being tested. Many researchers believed that models would improve at the same rate as long as they processed more data while using more specialized AI chips, but those two factors don’t seem to be enough.”
“The issue is particularly concerning for Google, as Gemini models have lagged OpenAI’s GPT models in terms of the number of developers and customers who use them. Google has been hoping its advantage over OpenAI in computing resources could help it leapfrog OpenAI’s models in terms of quality. In the meantime, both companies are developing new products, powered by existing versions of the technology, that could automate the tedious or complex work of software programmers and other office workers.”
This is an issue that Google is focused on for its developers using its Gemini AI models and tools, as I’ve discussed.
Google of course has a response:
“We’re pleased with the progress we’re seeing on Gemini and we’ll share more when we’re ready,” a Google spokesperson said. The spokesperson said the company is rethinking how it approaches training data and “investing significantly” in data. Google has also succeeded at speeding up how quickly its models can produce answers, which is “important for serving AI at Google’s scale,” the spokesperson said.”
And Google is lockstep in developing AI reasoning and agentic capabilities for its models:
“At OpenAI, researchers have invented new techniques such as reasoning models to make up for the slowdown in advancements that used traditional scaling law techniques during the model training phase.”
“Google appears to be following suit. In recent weeks, DeepMind has staffed a team within its Gemini unit, led by principal research scientist Jack Rae and former Character.AI co-founder Noam Shazeer, that aims to develop similar capabilities.”
“Researchers at DeepMind, the Google unit developing Gemini, also have been focusing on making manual improvements to the models. Those include changing their “hyperparameters,” or variables that determine how the model processes information, such as how quickly it draws connections between different concepts or patterns within training data, said the person who is involved in the work. Researchers test different hyperparameters during a process called model tuning to see which variables lead to the best results.”
“Google may not be starting from scratch, however. Google researchers previously invented a key technique behind OpenAI’s reasoning models, though one of the Google researchers decamped to OpenAI to work on the technique there.”
And Google is focused on AI synthetic data capabilities as well as multimodal AI innovations:
“Researchers had also hoped that using AI-generated data, also known as synthetic data, as well as audio and video as part of the Gemini training data could lead to significant improvements, but those factors don’t appear to have made a major impact, this person said. (Gemini models are “demonstrating strong performance with information, and we continue to explore and advance multimodal capabilities,” the spokesperson said.)”
“OpenAI and other developers also use synthetic data but have found they are limited in how much they can improve AI models.”
This is an issue that’s also well debated in this AI Tech Wave, as I’ve discussed at length.
Overall, as I said in ‘Part 1’ of this AI Scaling debate:
“Scaling AI is going to come in all forms of AI processes and sizes.”
“We need to expand our mental frameworks accordingly. OpenAI Sam Altman’s compass is pointing in the right direction. AGI however defined, is the general destination.”
“The paths to the destination via AI Scaling with massive Compute, may shift, fork, twist, and turn. But the destination remains the same.”
“OpenAI, Anthropic and others are figuring it out with flexibility.”
Add Google to this list as well. Stay tuned.
(NOTE: The discussions here are for information purposes only, and not meant as investment advice at any time. Thanks for joining us here)