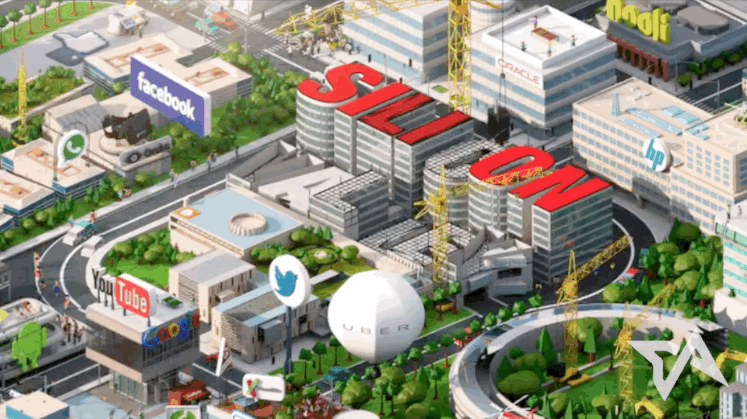
AI: Smaller AI Startups doing more with less people. RTZ #640
The Bigger Picture, Sunday, 2/23/25
I’ve discussed one of the key lessons from DeepSeek’s recent ‘overnight success’, was how it managed to do so ‘much more with less’. Not just the constraints of not having access to the latest AI chips from Nvidia due to US/China tech/AI curbs, but doing it all with far less capital and employees. Less than 200 by most measures. This lesson has already being taken to heart by tech/AI startups in this AI Tech Wave. And that is the Bigger Picture I’d like to discuss this sunday.
The New York Times lays it out well in “A.I. Is Changing How Silicon Valley Builds Start-Ups”:
“Tech start-ups typically raised huge sums to hire armies of workers and grow fast. Now artificial intelligence tools are making workers more productive and spurring tales of “tiny team” success.”
“The old Silicon Valley model dictated that start-ups should raise a huge sum of money from venture capital investors and spend it hiring an army of employees to scale up fast. Profits would come much later. Until then, head count and fund-raising were badges of honor among founders, who philosophized that bigger was better.”
The NYT tells the story of Gamma, an AI startup that is doing far more with far fewer employees as its stage of growth with its AI tools for employee productivity:
“But Gamma is among a growing cohort of start-ups, most of them working on A.I. products, that are also using A.I. to maximize efficiency. They make money and are growing fast without the funding or employees they would have needed before. The biggest bragging rights for these start-ups are for making the most revenue with the fewest workers.”
“Stories of “tiny team” success have now become a meme, with techies excitedly sharing lists that show how Anysphere, a start-up that makes the coding software Cursor, hit $100 million in annual recurring revenue in less than two years with just 20 employees, and how ElevenLabs, an A.I. voice start-up, did the same with around 50 workers.”
The meme being referred to by Ben Lang, summarizes this ‘tiny teams’ approach with examples of AI startups:
“Tiny teams are the future:
• Cursor: 0 to $100M ARR in 21 months w/ 20 people
• Bolt: 0 to $20M ARR in 2 months w/ 15 people
• Lovable: 0 to $10M ARR in 2 months w/ 15 people
• Mercor: 0 to $50M ARR in 2 years w/ 30 people
• ElevenLabs: 0 to $100M ARR in 2 years w/ 50 people”
The NY Times piece provides further context:
“With A.I. tools, some start-ups are now declaring that they will stop hiring at a certain size. Runway Financial, a finance software company, has said it plans to top out at 100 employees because each of its workers will do the work of 1.5 people. Agency, a start-up using A.I. for customer service, also plans to hire no more than 100 workers.”
“It’s about eliminating roles that are not necessary when you have smaller teams,” said Elias Torres, Agency’s founder.”
And DeepSeek of course is the overseas, open source poster child for this small teams approach:
“The idea of A.I.-driven efficiency was bolstered last month by DeepSeek, the Chinese A.I. start-up that showed it could build A.I. tools for a small fraction of the typical cost. Its breakthrough, built on open source tools that are freely available online, set off an explosion of companies building new products using DeepSeek’s inexpensive techniques.”
“DeepSeek was a watershed moment,” said Gaurav Jain, an investor at the venture firm Afore Capital, which has backed Gamma. “The cost of compute is going to go down very, very fast, very quickly.”
This trend of course echoes with the history of silicon valley, particularly since Amazon AWS in the 2000s gave tech startups to also do far more with less early on:
“Mr. Jain compared new A.I. start-ups to the wave of companies that arose in the late 2000s, after Amazon began offering cheap cloud computing services. That lowered the cost of starting a company, leading to a flurry of new start-ups that could be built more cheaply.”
“Before this A.I. boom, start-ups generally burned $1 million to get to $1 million in revenue, Mr. Jain said. Now getting to $1 million in revenue costs one-fifth as much and could eventually drop to one-tenth, according to an analysis of 200 start-ups conducted by Afore.”
“This time we’re automating humans as opposed to just the data centers,” Mr. Jain said.”
Which raises the question now as it did then, of potential challenges for VCs:
“But if start-ups can become profitable without spending much, that could become a problem for venture capital investors, who allocate tens of billions to invest in A.I. start-ups. Last year, A.I. companies raised $97 billion in funding, making up 46 percent of all venture investment in the United States, according to PitchBook, which tracks start-ups.”
“Venture capital only works if you get money into the winners,” said Terrence Rohan, an investor with Otherwise Fund, which focuses on very young start-ups. He added, “If the winner of the future needs a lot less money because they’ll have a lot less people, how does that change V.C.?”
I’d venture to say that VCs will still have a key role to play, especially after the startups find their product market fit, and ramp up for global markets of billions of mainstream users in the growth ramp up stage.
To be clear, this smaller team trend is distinct from the opportunities for AI/Tech startups for Small AI applications vs LLM AI based models. That is a separate trend I’ve discussed at length.
But there’s no question of opportunities for tech startups to be smaller on the way to early success right now in this AI Tech Wave, To carry on the silicon valley tradition of doing ‘more with less’.
And that is a notable Bigger Picture to keep in mind this time around. Stay tuned.
(NOTE: The discussions here are for information purposes only, and not meant as investment advice at any time. Thanks for joining us here)