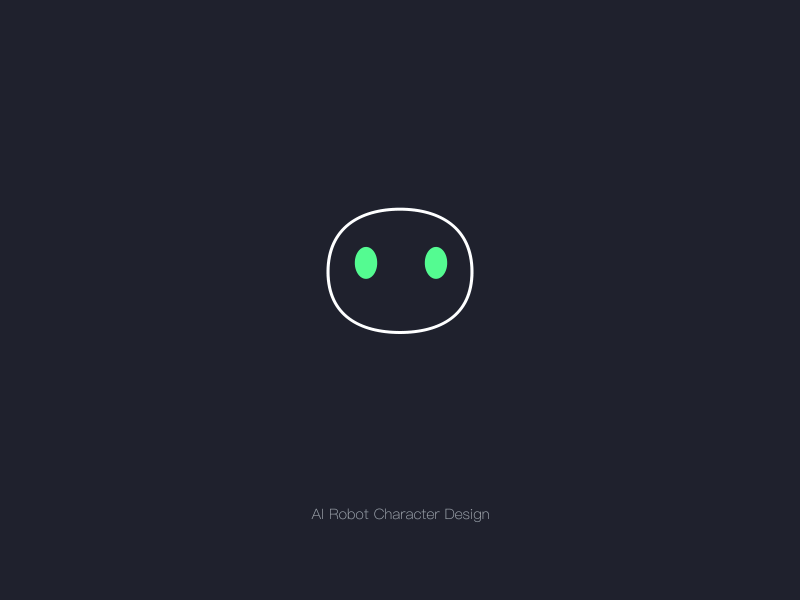
AI: Managing AI quality vs costs. RTZ #399
Yesterday, I discussed the fast-growing market for customizable AI chatbots that can be customized by millions of users to be used as ‘smart agents’ and ‘companions’. Companies like Meta, Character.ai, Replika, and recently Google amongst others are racing to provide these AI chatbots to cater to the emotional needs of users. This would be in contrast with the regular AI chatGPT type services and related ‘Reasoning’, ‘Agentic’ software that cater to the rational side of their work and personal lives. While maintaining user trust that their privacy and safety are prioritized.
The conversational AI services and ‘agent/companions’ serving both types of uses run massive amounts of AI Inference compute, where they take massive amounts of user data and prompts and run them against the underlying LLM AI models trained at for months or years, at costs in the billions of dollars. These inference comute costs run up quite the ‘variable cost’ bill for the companies providing the services, than then have to be passed onto customers, or paid for with advertising or other revenue streams down the road.
And in an attempt to mitigate and reduce these inference costs, these companies are constantly tweaking their training and inference architectures to meaningfully reduce their variable operating costs against massive amounts of still scarce AI GPU data center Compute, using high cost Nvidia AI chips and infrastructure, and of course cloud data center services like Amazon AWS, Microsoft Azure, Google Cloud and many others.
So as these companies meaningfully improve their underlying technologies to improve operating efficiencies, it can affect how the upstream AI applications end up performing. And to consumer users, this comes across as overnight, startling changes in the ‘personalities’ and capabilities of their AI chatbots.
An illustrative case in point is what Character.ai, a leading provider of customizable AI chatbots and companions, is going through following recent technical changes to their operations. As 404 Media reports in “‘No Bot is Themselves Anymore:’ Character.ai Users Report Sudden Personality Changes to Chatbots”:
“Users of massively popular AI chatbot platform Character.AI are reporting that their bots’ personalities have changed, that they aren’t responding to romantic roleplay prompts, are responding curtly, are not as “smart” as they formerly were, or are requiring a lot more effort to develop storylines or meaningful responses.”
“Character.AI, which is valued at $1 billion after raising $150 million in a round led by a16z last year, allows users to create their own characters with personalities and backstories, or choose from a vast library of bots that other people, or the company itself, has created.”
“In the r/CharacterAI subreddit, where users trade advice and stories related to the chatbot platform, many users are complaining that their bots aren’t as good as they used to be. Most say they noticed a change recently, in the last few days to weeks.”
They then go on to outline the company’s response to these customer complaints:
“In a company blog post last week, Character.AI claimed that it serves “around 20,000 queries per second–about 20% of the request volume served by Google Search, according to public sources.” It serves that volume at a cost of less than one cent per hour of conversation, it said. “
“The platform is free to use with limited features, and users can sign up for a subscription to access faster messages and early access to new features for $9.99 a month.”
“I asked Character.AI whether it’s changed anything that would cause the issues users are reporting. “We haven’t made any major changes. So I’m not sure why some users are having the experience you describe,” a spokesperson for the company said in an email. “However, here is one possibility: Like most B2C platforms, we’re always running tests on feature tweaks to continually improve our user experience. So it’s conceivable that some users encountered a test environment that behaved a bit differently than they’re used to. Feedback that some of them may be finding it more difficult to have conversations is valuable for us, and we’ll share it with our product team.”
Explaining these changes is tough, something I’ve discussed before in AI systems. Digging deeper into Character.ai’s blog post, we get more details on what may have affected user experiences:
“At Character.AI, we’re building toward AGI. In that future state, large language models (LLMs) will enhance daily life, providing business productivity and entertainment and helping people with everything from education to coaching, support, brainstorming, creative writing and more.
“To make that a reality globally, it’s critical to achieve highly efficient “inference” – the process by which LLMs generate replies. As a full-stack AI company, Character.AI designs its model architecture, inference stack and product from the ground up. And we’re excited to share that we have made a number of breakthroughs in inference technology – breakthroughs that will make LLMs easier and more cost-effective to scale to a global audience.”
“Our inference innovations are described in a technical blog post released today and available here. In short: Character.AI serves around 20,000 queries per second – about 20% of the request volume served by Google Search, according to public sources. We manage to serve that volume at a cost of less than one cent per hour of conversation. We are able to do so because of our innovations around Transformer architecture and “attention KV cache” – the amount of data stored and retrieved during LLM text generation – and around improved techniques for inter-turn caching.”
“These innovations, taken together, make serving consumer LLMs much more efficient than with legacy technology. Since we launched Character.AI in 2022, we have reduced our serving costs by at least 33X. It now costs us 13.5 times less to serve our traffic than it would cost a competitor building on top of the most efficient leading commercial APIs.”
“These efficiencies clear a path to serving LLMs at a massive scale. Assume, for example, a future state where an AI company is serving 100 million daily active users, each of whom uses the service for an hour per day. At that scale, with serving costs of $0.01 per hour, the company would spend $365 million per year – i.e., $3.65 per daily active user per year – on serving costs. By contrast, a competitor using leading commercial APIs would spend at least $4.75 billion. Continued efficiency improvements and economies of scale will no doubt lower those numbers for both us and competitors, but these numbers illustrate the importance of our inference improvements from a business perspective.”
“Put another way, these sorts of inference improvements unlock the ability to create a profitable B2C AI business at scale. We are excited to continue to iterate on them, in service of our mission to make our exciting technology available to consumers around the world to use throughout their day.”
There’s a lot more useful information in the technical blog post as well for those interested.
But the high-level takeaway of note is that the company is meaningfully focused on improving their AI serving costs by over 30 times, while processing daily data flows and queries a fifth of Google’s almost 10 billion search queries a day. And that in a business that is still trying to find its ultimate ‘product-market-fit’ at Scale. And as competitors like Meta, and potentially Google are ramping up to serve similar products in to billions of their mainstream users.
And these architectural and operational improvements are by definition going to change how the AI products behave, since every prompt query and ‘conversation’ is the result of billions of unique calculations per second, for every user on massive amounts of probabilistic matrix math computations on ever-changing user Data flows. So no AI result is going to be similar to the previous or next one. And tthe results themselves are fluid and barely explainable by the engineers who create and maintain these systems.
AI applications and services have the balancing act of most businesses between cost/efficiencies and product quality maintenance, but at EXTREME variations in scale at both ends.
And that is something that is going to be par for the course in this AI Tech Wave for at least the next 2-3 years, for most companies in the space large and small.
The Character.ai experience is a good case study of the challenges of balancing these two key priorities. A tough balancing act indeed. Stay tuned.
(NOTE: The discussions here are for information purposes only, and not meant as investment advice at any time. Thanks for joining us here)